Mapping outliers#
This notebook digs more into the outlier areas seen on the added utility maps of England and Wales plotted in the previous notebook.
Plain English summary#
A few areas in England and Wales break the trend shown by the rest. For the treated ischaemic population as a whole, most places show an improvement in added utility in the mothership scenario compared with drip-and-ship. However a few places show a worsening instead.
We will find out more about these outlier areas to see if we can find general trends of when the mothership scenario is a bad idea.
Aims#
Find the names of regions that break the trend
Look for trends in the treatment times of the outlier regions
Use existing generic geography and outcome matrix methods to check that the outlier results make sense.
Methods#
We define outlier regions as any that has better added utility in the drip-and-ship scenario than in mothership.
First we look for other regions that share similar travel times to the outliers.
Then we frame some of the outlier regions in the same context as the generic geography and the outcome matrix results to check what the stroke outcome model has output to create these outliers.
Import packages#
# import contextily as ctx
import geopandas
import matplotlib.pyplot as plt
import numpy as np
import pandas as pd
import os
import stroke_maps.load_data
import stroke_maps.catchment
import stroke_maps.geo # to make catchment area geometry
pd.set_option('display.max_rows', 150)
dir_output = 'output'
limit_to_england = False
Load data#
Load shape file#
lsoa_gdf = stroke_maps.load_data.lsoa_geography()
lsoa_gdf = lsoa_gdf.to_crs('EPSG:27700')
lsoa_gdf.head(3)
OBJECTID | LSOA11CD | LSOA11NM | LSOA11NMW | BNG_E | BNG_N | LONG | LAT | Shape__Area | Shape__Length | GlobalID | geometry | |
---|---|---|---|---|---|---|---|---|---|---|---|---|
0 | 1 | E01000001 | City of London 001A | City of London 001A | 532129 | 181625 | -0.09706 | 51.51810 | 157794.481079 | 1685.391778 | b12173a3-5423-4672-a5eb-f152d2345f96 | POLYGON ((532282.642 181906.500, 532248.262 18... |
1 | 2 | E01000002 | City of London 001B | City of London 001B | 532480 | 181699 | -0.09197 | 51.51868 | 164882.427628 | 1804.828196 | 90274dc4-f785-4afb-95cd-7cc1fc9a2cad | POLYGON ((532746.826 181786.896, 532248.262 18... |
2 | 3 | E01000003 | City of London 001C | City of London 001C | 532245 | 182036 | -0.09523 | 51.52176 | 42219.805717 | 909.223277 | 7e89d0ba-f186-45fb-961c-8f5ffcd03808 | POLYGON ((532293.080 182068.426, 532419.605 18... |
# Load country outline
# outline = geopandas.read_file('./data/Countries_(December_2022)_GB_BFC.zip')
if limit_to_england:
# mask = outline['CTRY22NM'] == 'England'
# outline = outline[mask]
outline = stroke_maps.load_data.england_outline()
else:
# mask = (outline['CTRY22NM'] == 'England') | (outline['CTRY22NM'] == 'Wales')
# outline = outline[mask]
outline = stroke_maps.load_data.englandwales_outline()
outline
country | OBJECTID | ctry11cd | ctry11cdo | ctry11nm | ctry11nmw | GlobalID | geometry | |
---|---|---|---|---|---|---|---|---|
0 | 0 | 1 | E92000001 | 921 | England | Lloegr | 27bbf7ca-99bd-4fe8-87a1-d498d48e3084 | MULTIPOLYGON (((83994.599 5397.099, 84001.300 ... |
Load hospital info#
Load in the stroke unit coordinates and merge in the services information:
df_units = stroke_maps.load_data.stroke_unit_region_lookup()
df_units.head(3).T
postcode | SY231ER | CB20QQ | L97AL |
---|---|---|---|
stroke_team | Bronglais Hospital (Aberystwyth) | Addenbrooke's Hospital, Cambridge | University Hospital Aintree, Liverpool |
short_code | AB | AD | AI |
ssnap_name | Bronglais Hospital | Addenbrooke's Hospital | University Hospital Aintree |
use_ivt | 1 | 1 | 1 |
use_mt | 0 | 1 | 1 |
use_msu | 0 | 1 | 1 |
transfer_unit_postcode | nearest | nearest | nearest |
lsoa | Ceredigion 002A | Cambridge 013D | Liverpool 005A |
lsoa_code | W01000512 | E01017995 | E01006654 |
region | Hywel Dda University Health Board | NHS Cambridgeshire and Peterborough ICB - 06H | NHS Cheshire and Merseyside ICB - 99A |
region_code | W11000025 | E38000260 | E38000101 |
region_type | LHB | SICBL | SICBL |
country | Wales | England | England |
icb | NaN | NHS Cambridgeshire and Peterborough Integrated... | NHS Cheshire and Merseyside Integrated Care Board |
icb_code | NaN | E54000056 | E54000008 |
isdn | NaN | East of England (South) | Cheshire and Merseyside |
hospitals_gdf = stroke_maps.load_data.stroke_unit_coordinates()
hospitals_gdf = pd.merge(
hospitals_gdf, df_units[['use_ivt', 'use_mt']],
left_index=True, right_index=True, how='right'
)
hospitals_gdf.head(3)
BNG_E | BNG_N | Latitude | Longitude | geometry | use_ivt | use_mt | |
---|---|---|---|---|---|---|---|
postcode | |||||||
SY231ER | 259208 | 281805 | 52.416068 | -4.071578 | POINT (259208.000 281805.000) | 1 | 0 |
CB20QQ | 546375 | 254988 | 52.173741 | 0.139114 | POINT (546375.000 254988.000) | 1 | 1 |
L97AL | 338020 | 397205 | 53.467918 | -2.935131 | POINT (338020.000 397205.000) | 1 | 1 |
Load LSOA model output data#
lsoa_data = pd.read_csv(os.path.join(dir_output, 'cohort_outcomes_weighted.csv'))
lsoa_data.head(3).T
0 | 1 | 2 | |
---|---|---|---|
lsoa | Adur 001A | Adur 001B | Adur 001C |
closest_ivt_time | 17.6 | 18.7 | 17.6 |
closest_ivt_unit | BN25BE | BN25BE | BN112DH |
closest_mt_time | 17.6 | 18.7 | 19.8 |
closest_mt_unit | BN25BE | BN25BE | BN25BE |
transfer_mt_time | 0.0 | 0.0 | 31.6 |
transfer_mt_unit | BN25BE | BN25BE | BN25BE |
mt_transfer_required | False | False | True |
ivt_drip_ship | 107.6 | 108.7 | 107.6 |
mt_drip_ship | 167.6 | 168.7 | 259.2 |
ivt_mothership | 107.6 | 108.7 | 109.8 |
mt_mothership | 167.6 | 168.7 | 169.8 |
drip_ship_nlvo_ivt_added_utility | 0.11685 | 0.11641 | 0.11685 |
drip_ship_nlvo_ivt_mean_mrs | 1.63653 | 1.63913 | 1.63653 |
drip_ship_nlvo_ivt_mrs_less_equal_2 | 0.70649 | 0.706 | 0.70649 |
drip_ship_nlvo_ivt_mrs_shift | -0.64347 | -0.64087 | -0.64347 |
drip_ship_nlvo_ivt_added_mrs_less_equal_2 | 0.12649 | 0.126 | 0.12649 |
drip_ship_lvo_ivt_added_utility | 0.05872 | 0.05841 | 0.05872 |
drip_ship_lvo_ivt_mean_mrs | 3.34655 | 3.34823 | 3.34655 |
drip_ship_lvo_ivt_mrs_less_equal_2 | 0.32879 | 0.32847 | 0.32879 |
drip_ship_lvo_ivt_mrs_shift | -0.29345 | -0.29177 | -0.29345 |
drip_ship_lvo_ivt_added_mrs_less_equal_2 | 0.06379 | 0.06347 | 0.06379 |
drip_ship_lvo_ivt_mt_added_utility | 0.16361 | 0.16295 | 0.10928 |
drip_ship_lvo_ivt_mt_mean_mrs | 2.81136 | 2.81494 | 3.10174 |
drip_ship_lvo_ivt_mt_mrs_less_equal_2 | 0.43807 | 0.43736 | 0.37981 |
drip_ship_lvo_ivt_mt_mrs_shift | -0.82864 | -0.82506 | -0.53826 |
drip_ship_lvo_ivt_mt_added_mrs_less_equal_2 | 0.17307 | 0.17236 | 0.11481 |
drip_ship_lvo_mt_added_utility | 0.16361 | 0.16295 | 0.10928 |
drip_ship_lvo_mt_mean_mrs | 2.81136 | 2.81494 | 3.10174 |
drip_ship_lvo_mt_mrs_less_equal_2 | 0.43807 | 0.43736 | 0.37981 |
drip_ship_lvo_mt_mrs_shift | -0.82864 | -0.82506 | -0.53826 |
drip_ship_lvo_mt_added_mrs_less_equal_2 | 0.17307 | 0.17236 | 0.11481 |
mothership_nlvo_ivt_added_utility | 0.11685 | 0.11641 | 0.11596 |
mothership_nlvo_ivt_mean_mrs | 1.63653 | 1.63913 | 1.64173 |
mothership_nlvo_ivt_mrs_less_equal_2 | 0.70649 | 0.706 | 0.70551 |
mothership_nlvo_ivt_mrs_shift | -0.64347 | -0.64087 | -0.63827 |
mothership_nlvo_ivt_added_mrs_less_equal_2 | 0.12649 | 0.126 | 0.12551 |
mothership_lvo_ivt_added_utility | 0.05872 | 0.05841 | 0.05811 |
mothership_lvo_ivt_mean_mrs | 3.34655 | 3.34823 | 3.3499 |
mothership_lvo_ivt_mrs_less_equal_2 | 0.32879 | 0.32847 | 0.32815 |
mothership_lvo_ivt_mrs_shift | -0.29345 | -0.29177 | -0.2901 |
mothership_lvo_ivt_added_mrs_less_equal_2 | 0.06379 | 0.06347 | 0.06315 |
mothership_lvo_ivt_mt_added_utility | 0.16361 | 0.16295 | 0.16229 |
mothership_lvo_ivt_mt_mean_mrs | 2.81136 | 2.81494 | 2.81852 |
mothership_lvo_ivt_mt_mrs_less_equal_2 | 0.43807 | 0.43736 | 0.43664 |
mothership_lvo_ivt_mt_mrs_shift | -0.82864 | -0.82506 | -0.82148 |
mothership_lvo_ivt_mt_added_mrs_less_equal_2 | 0.17307 | 0.17236 | 0.17164 |
mothership_lvo_mt_added_utility | 0.16361 | 0.16295 | 0.16229 |
mothership_lvo_mt_mean_mrs | 2.81136 | 2.81494 | 2.81852 |
mothership_lvo_mt_mrs_less_equal_2 | 0.43807 | 0.43736 | 0.43664 |
mothership_lvo_mt_mrs_shift | -0.82864 | -0.82506 | -0.82148 |
mothership_lvo_mt_added_mrs_less_equal_2 | 0.17307 | 0.17236 | 0.17164 |
drip_ship_lvo_mix_added_utility | 0.15548 | 0.15485 | 0.10536 |
drip_ship_lvo_mix_mrs_less_equal_2 | 0.4296 | 0.42892 | 0.37586 |
drip_ship_lvo_mix_mrs_shift | -0.78717 | -0.78373 | -0.51929 |
drip_ship_lvo_mix_added_mrs_less_equal_2 | 0.1646 | 0.16392 | 0.11086 |
mothership_lvo_mix_added_utility | 0.15548 | 0.15485 | 0.15422 |
mothership_lvo_mix_mrs_less_equal_2 | 0.4296 | 0.42892 | 0.42823 |
mothership_lvo_mix_mrs_shift | -0.78717 | -0.78373 | -0.7803 |
mothership_lvo_mix_added_mrs_less_equal_2 | 0.1646 | 0.16392 | 0.16323 |
drip_ship_weighted_added_utility | 0.03392 | 0.03379 | 0.02764 |
drip_ship_weighted_mrs_less_equal_2 | 0.14107 | 0.14092 | 0.13432 |
drip_ship_weighted_mrs_shift | -0.17815 | -0.1774 | -0.14454 |
drip_ship_weighted_added_mrs_less_equal_2 | 0.03626 | 0.03611 | 0.02951 |
mothership_weighted_added_utility | 0.03392 | 0.03379 | 0.03366 |
mothership_weighted_mrs_less_equal_2 | 0.14107 | 0.14092 | 0.14077 |
mothership_weighted_mrs_shift | -0.17815 | -0.1774 | -0.17665 |
mothership_weighted_added_mrs_less_equal_2 | 0.03626 | 0.03611 | 0.03597 |
drip_ship_weighted_treated_added_utility | 0.13633 | 0.13579 | 0.11106 |
drip_ship_weighted_treated_mrs_less_equal_2 | 0.56689 | 0.5663 | 0.53979 |
drip_ship_weighted_treated_mrs_shift | -0.71592 | -0.7129 | -0.58086 |
drip_ship_weighted_treated_added_mrs_less_equal_2 | 0.14571 | 0.14512 | 0.11861 |
mothership_weighted_treated_added_utility | 0.13633 | 0.13579 | 0.13525 |
mothership_weighted_treated_mrs_less_equal_2 | 0.56689 | 0.5663 | 0.56571 |
mothership_weighted_treated_mrs_shift | -0.71592 | -0.7129 | -0.70988 |
mothership_weighted_treated_added_mrs_less_equal_2 | 0.14571 | 0.14512 | 0.14453 |
# Merge with shape file
lsoa_data_gdf = lsoa_gdf.merge(lsoa_data, left_on='LSOA11NM', right_on='lsoa', how='right')
lsoa_data_gdf.head()
OBJECTID | LSOA11CD | LSOA11NM | LSOA11NMW | BNG_E | BNG_N | LONG | LAT | Shape__Area | Shape__Length | ... | mothership_weighted_mrs_shift | mothership_weighted_added_mrs_less_equal_2 | drip_ship_weighted_treated_added_utility | drip_ship_weighted_treated_mrs_less_equal_2 | drip_ship_weighted_treated_mrs_shift | drip_ship_weighted_treated_added_mrs_less_equal_2 | mothership_weighted_treated_added_utility | mothership_weighted_treated_mrs_less_equal_2 | mothership_weighted_treated_mrs_shift | mothership_weighted_treated_added_mrs_less_equal_2 | |
---|---|---|---|---|---|---|---|---|---|---|---|---|---|---|---|---|---|---|---|---|---|
0 | 30557.0 | E01031349 | Adur 001A | Adur 001A | 524915.0 | 105607.0 | -0.22737 | 50.83651 | 3.641032e+05 | 3054.751704 | ... | -0.17815 | 0.03626 | 0.13633 | 0.56689 | -0.71592 | 0.14571 | 0.13633 | 0.56689 | -0.71592 | 0.14571 |
1 | 30558.0 | E01031350 | Adur 001B | Adur 001B | 524825.0 | 106265.0 | -0.22842 | 50.84244 | 2.921732e+05 | 2977.102897 | ... | -0.17740 | 0.03611 | 0.13579 | 0.56630 | -0.71290 | 0.14512 | 0.13579 | 0.56630 | -0.71290 | 0.14512 |
2 | 30559.0 | E01031351 | Adur 001C | Adur 001C | 523053.0 | 108004.0 | -0.25300 | 50.85845 | 5.281768e+06 | 11671.349143 | ... | -0.17665 | 0.03597 | 0.11106 | 0.53979 | -0.58086 | 0.11861 | 0.13525 | 0.56571 | -0.70988 | 0.14453 |
3 | 30560.0 | E01031352 | Adur 001D | Adur 001D | 524141.0 | 106299.0 | -0.23812 | 50.84290 | 2.452292e+05 | 2134.908586 | ... | -0.17665 | 0.03597 | 0.11106 | 0.53979 | -0.58086 | 0.11861 | 0.13525 | 0.56571 | -0.70988 | 0.14453 |
4 | 30578.0 | E01031370 | Adur 001E | Adur 001E | 523561.0 | 105916.0 | -0.24649 | 50.83958 | 2.402445e+05 | 2447.096939 | ... | -0.17665 | 0.03597 | 0.11159 | 0.54036 | -0.58379 | 0.11918 | 0.13525 | 0.56571 | -0.70988 | 0.14453 |
5 rows × 88 columns
cols = [c for c in lsoa_data_gdf.columns if (('lvo' in c) & ('nlvo' not in c) & ('utility' in c))]
lsoa_data_gdf[cols].head(10).T
0 | 1 | 2 | 3 | 4 | 5 | 6 | 7 | 8 | 9 | |
---|---|---|---|---|---|---|---|---|---|---|
drip_ship_lvo_ivt_added_utility | 0.05872 | 0.05841 | 0.05872 | 0.05872 | 0.05902 | 0.05872 | 0.05872 | 0.05872 | 0.05872 | 0.05872 |
drip_ship_lvo_ivt_mt_added_utility | 0.16361 | 0.16295 | 0.10928 | 0.10928 | 0.10992 | 0.10928 | 0.10928 | 0.10928 | 0.10928 | 0.10928 |
drip_ship_lvo_mt_added_utility | 0.16361 | 0.16295 | 0.10928 | 0.10928 | 0.10992 | 0.10928 | 0.10928 | 0.10928 | 0.10928 | 0.10928 |
mothership_lvo_ivt_added_utility | 0.05872 | 0.05841 | 0.05811 | 0.05811 | 0.05811 | 0.05841 | 0.05783 | 0.05752 | 0.05752 | 0.05752 |
mothership_lvo_ivt_mt_added_utility | 0.16361 | 0.16295 | 0.16229 | 0.16229 | 0.16229 | 0.16295 | 0.16169 | 0.16103 | 0.16103 | 0.16103 |
mothership_lvo_mt_added_utility | 0.16361 | 0.16295 | 0.16229 | 0.16229 | 0.16229 | 0.16295 | 0.16169 | 0.16103 | 0.16103 | 0.16103 |
drip_ship_lvo_mix_added_utility | 0.15548 | 0.15485 | 0.10536 | 0.10536 | 0.10598 | 0.10536 | 0.10536 | 0.10536 | 0.10536 | 0.10536 |
mothership_lvo_mix_added_utility | 0.15548 | 0.15485 | 0.15422 | 0.15422 | 0.15422 | 0.15485 | 0.15364 | 0.15301 | 0.15301 | 0.15301 |
Patient proportions:
patient_proportions = pd.read_csv(
os.path.join('..', 'england_wales', 'output', 'patient_proportions.csv'),
index_col=0, header=None).squeeze()
patient_proportions
0
haemorrhagic 0.13600
lvo_no_treatment 0.14648
lvo_ivt_only 0.00840
lvo_ivt_mt 0.08500
lvo_mt_only 0.01500
nlvo_no_treatment 0.50252
nlvo_ivt 0.10660
Name: 1, dtype: float64
# Proportion of treated ischaemic patients:
prop_ischaemic_treated = 0.0
for key, value in patient_proportions.items():
if (('lvo' in key) & ('no_treat' not in key)):
print(key)
prop_ischaemic_treated += value
prop_ischaemic_treated
lvo_ivt_only
lvo_ivt_mt
lvo_mt_only
nlvo_ivt
0.21500000000000002
prop_nlvo_of_treated = patient_proportions['nlvo_ivt'] / prop_ischaemic_treated
prop_lvo_of_treated = 1.0 - prop_nlvo_of_treated
prop_nlvo_of_treated, prop_lvo_of_treated
(0.49581395348837204, 0.5041860465116279)
Calculate difference between Mothership and Drip and Ship#
cohort_names = ['nlvo_ivt', 'lvo_mix', 'weighted_treated']
outcome_names = ['added_utility', 'added_mrs_less_equal_2', 'mrs_shift']
cols_diff = [f'{c}_{o}_mothership_minus_dripship' for c in cohort_names for o in outcome_names]
cols_moth = [f'mothership_{c}_{o}' for c in cohort_names for o in outcome_names]
cols_drip = [f'drip_ship_{c}_{o}' for c in cohort_names for o in outcome_names]
lsoa_data_gdf[cols_diff] = lsoa_data_gdf[cols_moth].values - lsoa_data_gdf[cols_drip].values
Map outliers#
Make a new dataframe with only a subset of the full data:
data_field = 'added_utility'
cols = [
f'nlvo_ivt_{data_field}_mothership_minus_dripship',
f'lvo_mix_{data_field}_mothership_minus_dripship',
f'weighted_treated_{data_field}_mothership_minus_dripship',
]
gdf_outliers = lsoa_data_gdf[['geometry'] + cols]
Find outliers separately for each case because the outlying condition is different each time.
For nLVO, most of the map has negative mothership minus drip-and-ship added utility, so pick out values that are positive.
For the LVO and ischaemic mix, most of the map is positive so pick out any negative values.
col = cols[0]
gdf_outliers[f'outlier_{col}'] = gdf_outliers[col] > 0.0
# How many values are outliers?
print(len(gdf_outliers.loc[gdf_outliers[f'outlier_{col}'] == True]))
0
/home/anna/miniconda3/lib/python3.9/site-packages/geopandas/geodataframe.py:1443: SettingWithCopyWarning:
A value is trying to be set on a copy of a slice from a DataFrame.
Try using .loc[row_indexer,col_indexer] = value instead
See the caveats in the documentation: https://pandas.pydata.org/pandas-docs/stable/user_guide/indexing.html#returning-a-view-versus-a-copy
super().__setitem__(key, value)
col = cols[1]
gdf_outliers[f'outlier_{col}'] = gdf_outliers[col] < 0.0
# How many values are outliers?
print(len(gdf_outliers.loc[gdf_outliers[f'outlier_{col}'] == True]))
0
/home/anna/miniconda3/lib/python3.9/site-packages/geopandas/geodataframe.py:1443: SettingWithCopyWarning:
A value is trying to be set on a copy of a slice from a DataFrame.
Try using .loc[row_indexer,col_indexer] = value instead
See the caveats in the documentation: https://pandas.pydata.org/pandas-docs/stable/user_guide/indexing.html#returning-a-view-versus-a-copy
super().__setitem__(key, value)
col = cols[2]
gdf_outliers[f'outlier_{col}'] = gdf_outliers[col] < 0.0
# How many values are outliers?
print(len(gdf_outliers.loc[gdf_outliers[f'outlier_{col}'] == True]))
266
/home/anna/miniconda3/lib/python3.9/site-packages/geopandas/geodataframe.py:1443: SettingWithCopyWarning:
A value is trying to be set on a copy of a slice from a DataFrame.
Try using .loc[row_indexer,col_indexer] = value instead
See the caveats in the documentation: https://pandas.pydata.org/pandas-docs/stable/user_guide/indexing.html#returning-a-view-versus-a-copy
super().__setitem__(key, value)
Only the mixed population has outliers. Plot their locations:
col = cols[2]
# Plot data
fig, ax = plt.subplots(figsize=(8, 10))
mask = gdf_outliers[f'outlier_{col}'] == True
print(len(gdf_outliers.loc[mask]))
gdf_outliers.loc[mask].plot(
ax=ax, # Set which axes to use for plot (only one here)
color='LimeGreen', # Column to apply colour
antialiased=False, # Avoids artifact boundry lines
edgecolor='face', # Make LSOA boundry same colour as area
)
# Add country border
outline.plot(ax=ax, edgecolor='k', facecolor='None', linewidth=1.0)
plt.show()
266
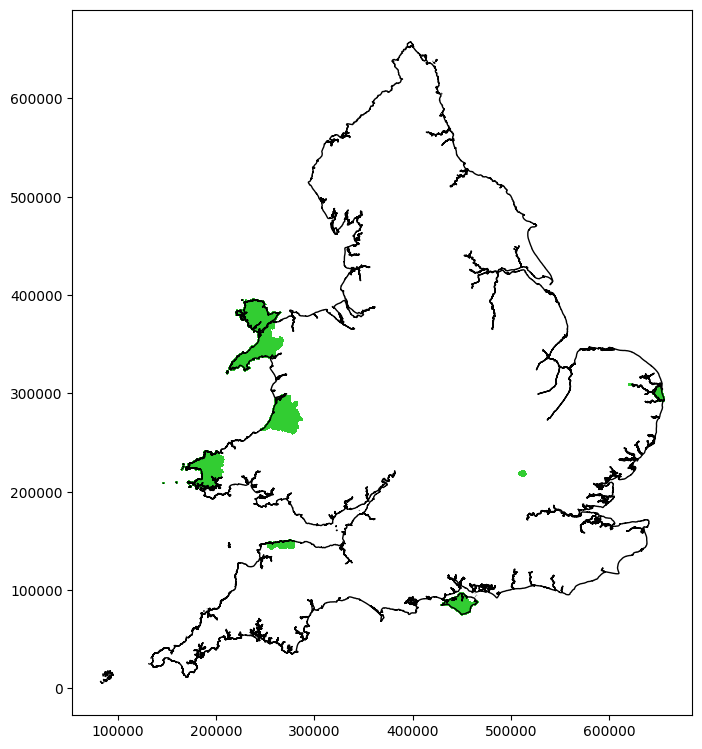
Check outlier times#
Make a smaller dataframe of all of the columns for just these outlier areas:
col = cols[2]
mask = gdf_outliers[f'outlier_{col}'] == True
gdf_out_adv = lsoa_data_gdf.loc[mask].copy()
gdf_out_adv
OBJECTID | LSOA11CD | LSOA11NM | LSOA11NMW | BNG_E | BNG_N | LONG | LAT | Shape__Area | Shape__Length | ... | mothership_weighted_treated_added_mrs_less_equal_2 | nlvo_ivt_added_utility_mothership_minus_dripship | nlvo_ivt_added_mrs_less_equal_2_mothership_minus_dripship | nlvo_ivt_mrs_shift_mothership_minus_dripship | lvo_mix_added_utility_mothership_minus_dripship | lvo_mix_added_mrs_less_equal_2_mothership_minus_dripship | lvo_mix_mrs_shift_mothership_minus_dripship | weighted_treated_added_utility_mothership_minus_dripship | weighted_treated_added_mrs_less_equal_2_mothership_minus_dripship | weighted_treated_mrs_shift_mothership_minus_dripship | |
---|---|---|---|---|---|---|---|---|---|---|---|---|---|---|---|---|---|---|---|---|---|
6195 | 17053.0 | E01017564 | Central Bedfordshire 033D | Central Bedfordshire 033D | 511088.0 | 218265.0 | -0.388520 | 51.85194 | 1.794032e+07 | 20786.770830 | ... | 0.10599 | -0.02931 | -0.03210 | 0.16838 | 0.01779 | 0.01888 | -0.09336 | -0.00556 | -0.00640 | 0.03641 |
6196 | 33344.0 | W01000523 | Ceredigion 001C | Ceredigion 001C | 268865.0 | 290703.0 | -3.933180 | 52.49843 | 1.294319e+08 | 55283.856672 | ... | 0.08350 | -0.04997 | -0.05460 | 0.28511 | 0.03201 | 0.03184 | -0.15103 | -0.00863 | -0.01102 | 0.06522 |
6197 | 33367.0 | W01000548 | Ceredigion 001D | Ceredigion 001D | 261295.0 | 285094.0 | -4.042280 | 52.44616 | 1.452597e+07 | 21925.550573 | ... | 0.08350 | -0.05255 | -0.05744 | 0.30018 | 0.02998 | 0.02971 | -0.14004 | -0.01094 | -0.01350 | 0.07823 |
6198 | 33368.0 | W01000549 | Ceredigion 001E | Ceredigion 001E | 267387.0 | 283780.0 | -3.952180 | 52.43587 | 2.582152e+07 | 27673.339921 | ... | 0.08291 | -0.05220 | -0.05705 | 0.29800 | 0.03004 | 0.02977 | -0.14047 | -0.01074 | -0.01328 | 0.07694 |
6199 | 34732.0 | W01001937 | Ceredigion 001F | Ceredigion 001F | 261990.0 | 288178.0 | -4.033340 | 52.47405 | 2.536853e+07 | 28049.044543 | ... | 0.08291 | -0.04958 | -0.05416 | 0.28268 | 0.03210 | 0.03192 | -0.15161 | -0.00840 | -0.01076 | 0.06372 |
... | ... | ... | ... | ... | ... | ... | ... | ... | ... | ... | ... | ... | ... | ... | ... | ... | ... | ... | ... | ... | ... |
32026 | 29482.0 | E01030263 | Waveney 005B | Waveney 005B | 653306.0 | 293630.0 | 1.729125 | 52.48177 | 7.875654e+05 | 3912.339748 | ... | 0.09557 | -0.04037 | -0.04419 | 0.23137 | 0.03527 | 0.03697 | -0.18337 | -0.00224 | -0.00327 | 0.02227 |
32027 | 29489.0 | E01030270 | Waveney 005C | Waveney 005C | 652508.0 | 293295.0 | 1.717146 | 52.47913 | 5.150318e+05 | 3402.978540 | ... | 0.09676 | -0.03940 | -0.04313 | 0.22592 | 0.03649 | 0.03829 | -0.18986 | -0.00114 | -0.00208 | 0.01629 |
32032 | 29487.0 | E01030268 | Waveney 006C | Waveney 006C | 651334.0 | 293510.0 | 1.700058 | 52.48160 | 3.534155e+05 | 4242.495260 | ... | 0.09557 | -0.03997 | -0.04374 | 0.22901 | 0.03581 | 0.03754 | -0.18620 | -0.00177 | -0.00276 | 0.01967 |
32033 | 29488.0 | E01030269 | Waveney 006D | Waveney 006D | 651416.0 | 293083.0 | 1.700941 | 52.47773 | 8.158435e+05 | 4654.804663 | ... | 0.09616 | -0.03904 | -0.04272 | 0.22370 | 0.03702 | 0.03882 | -0.19255 | -0.00069 | -0.00161 | 0.01383 |
32035 | 29466.0 | E01030247 | Waveney 007A | Waveney 007A | 655201.0 | 293800.0 | 1.757099 | 52.48241 | 9.738185e+05 | 6457.751416 | ... | 0.09557 | -0.03997 | -0.04374 | 0.22901 | 0.03581 | 0.03754 | -0.18620 | -0.00177 | -0.00276 | 0.01967 |
266 rows × 97 columns
Pick out the first part of the LSOA names to get an idea of their towns or regions:
sorted(list(set([s[:-5] for s in gdf_out_adv['LSOA11NM'].values])))
['Central Bedfordshire',
'Ceredigion',
'Great Yarmouth',
'Gwynedd',
'Isle of Anglesey',
'Isle of Wight',
'North Devon',
'Norwich',
'Pembrokeshire',
'Waveney']
Eyeball the travel and treatment time columns:
cols_to_check = [
'LSOA11NM',
'closest_ivt_time',
'closest_ivt_unit',
'closest_mt_time',
'closest_mt_unit',
'transfer_mt_time',
'transfer_mt_unit',
'mt_transfer_required',
'ivt_drip_ship',
'mt_drip_ship',
'ivt_mothership',
'mt_mothership'
]
gdf_out_adv[cols_to_check].T
6195 | 6196 | 6197 | 6198 | 6199 | 6200 | 6201 | 6202 | 6203 | 6204 | ... | 32021 | 32022 | 32023 | 32024 | 32025 | 32026 | 32027 | 32032 | 32033 | 32035 | |
---|---|---|---|---|---|---|---|---|---|---|---|---|---|---|---|---|---|---|---|---|---|
LSOA11NM | Central Bedfordshire 033D | Ceredigion 001C | Ceredigion 001D | Ceredigion 001E | Ceredigion 001F | Ceredigion 002A | Ceredigion 002B | Ceredigion 002D | Ceredigion 002F | Ceredigion 003A | ... | Waveney 004C | Waveney 004D | Waveney 004E | Waveney 004F | Waveney 005A | Waveney 005B | Waveney 005C | Waveney 006C | Waveney 006D | Waveney 007A |
closest_ivt_time | 21.9 | 16.5 | 10.1 | 12.2 | 18.7 | 6.9 | 6.9 | 7.9 | 6.9 | 9.0 | ... | 17.6 | 17.6 | 16.5 | 17.6 | 18.7 | 15.5 | 15.5 | 16.5 | 17.6 | 16.5 |
closest_ivt_unit | LU40DZ | SY231ER | SY231ER | SY231ER | SY231ER | SY231ER | SY231ER | SY231ER | SY231ER | SY231ER | ... | NR316LA | NR316LA | NR316LA | NR316LA | NR316LA | NR316LA | NR316LA | NR316LA | NR316LA | NR316LA |
closest_mt_time | 91.7 | 133.6 | 133.6 | 134.7 | 134.7 | 134.7 | 135.8 | 136.8 | 134.7 | 136.8 | ... | 111.1 | 111.1 | 112.1 | 111.1 | 110.0 | 111.1 | 108.9 | 111.1 | 110.0 | 111.1 |
closest_mt_unit | NW12BU | ST46QG | CF144XW | CF144XW | ST46QG | CF144XW | CF144XW | CF144XW | CF144XW | CF144XW | ... | CB20QQ | CB20QQ | CB20QQ | CB20QQ | CB20QQ | CB20QQ | CB20QQ | CB20QQ | CB20QQ | CB20QQ |
transfer_mt_time | 45.5 | 135.8 | 135.8 | 135.8 | 135.8 | 135.8 | 135.8 | 135.8 | 135.8 | 135.8 | ... | 105.7 | 105.7 | 105.7 | 105.7 | 105.7 | 105.7 | 105.7 | 105.7 | 105.7 | 105.7 |
transfer_mt_unit | NW12BU | CF144XW | CF144XW | CF144XW | CF144XW | CF144XW | CF144XW | CF144XW | CF144XW | CF144XW | ... | CB20QQ | CB20QQ | CB20QQ | CB20QQ | CB20QQ | CB20QQ | CB20QQ | CB20QQ | CB20QQ | CB20QQ |
mt_transfer_required | True | True | True | True | True | True | True | True | True | True | ... | True | True | True | True | True | True | True | True | True | True |
ivt_drip_ship | 111.9 | 106.5 | 100.1 | 102.2 | 108.7 | 96.9 | 96.9 | 97.9 | 96.9 | 99.0 | ... | 107.6 | 107.6 | 106.5 | 107.6 | 108.7 | 105.5 | 105.5 | 106.5 | 107.6 | 106.5 |
mt_drip_ship | 277.4 | 362.3 | 355.9 | 358.0 | 364.5 | 352.7 | 352.7 | 353.7 | 352.7 | 354.8 | ... | 333.3 | 333.3 | 332.2 | 333.3 | 334.4 | 331.2 | 331.2 | 332.2 | 333.3 | 332.2 |
ivt_mothership | 181.7 | 223.6 | 223.6 | 224.7 | 224.7 | 224.7 | 225.8 | 226.8 | 224.7 | 226.8 | ... | 201.1 | 201.1 | 202.1 | 201.1 | 200.0 | 201.1 | 198.9 | 201.1 | 200.0 | 201.1 |
mt_mothership | 241.7 | 283.6 | 283.6 | 284.7 | 284.7 | 284.7 | 285.8 | 286.8 | 284.7 | 286.8 | ... | 261.1 | 261.1 | 262.1 | 261.1 | 260.0 | 261.1 | 258.9 | 261.1 | 260.0 | 261.1 |
12 rows × 266 columns
Find the difference in travel times between LSOA to MT unit and LSOA to IVT unit.
gdf_out_adv['unit_time_diff'] = gdf_out_adv['closest_mt_time'] - gdf_out_adv['closest_ivt_time']
plt.scatter(np.arange(len(gdf_out_adv)), sorted(gdf_out_adv['unit_time_diff']))
plt.xlabel('Position in the list')
plt.ylabel('Difference in travel time')
plt.show()
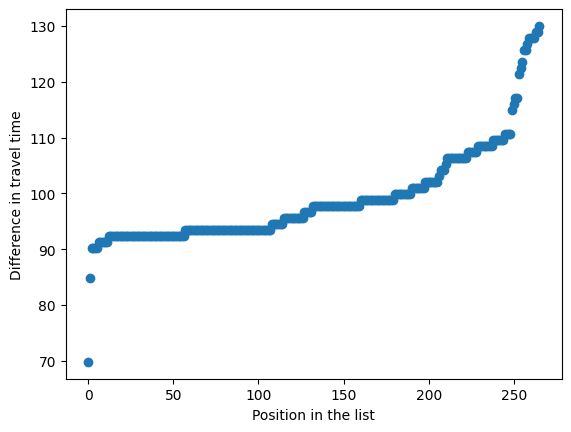
Eyeballing this, nearly all of the outliers have a difference in time of at least 90 minutes or an hour and a half.
The shortest time is for Central Bedfordshire, which is anomalous in the travel times matrix because none of its surrounding LSOAs have similar travel times. This LSOA has a longer time direct to the MT unit (91.7 mins) than the sum of the travel time to the IVT unit and then transfer to the MT unit (21.9 + 45.5 = 67.4 mins).
Find the shortest time excluding that LSOA:
mask_bed = gdf_out_adv['LSOA11NM'].str.contains('Bedfordshire')
gdf_out_adv.loc[~mask_bed, 'unit_time_diff'].min()
84.80000000000001
Pick out LSOAs similar to the outliers#
Find if there are other LSOAs in the full dataframe that are not flagged as outliers but have a similar difference in unit travel times.
lsoa_data_gdf['unit_time_diff'] = lsoa_data_gdf['closest_mt_time'] - lsoa_data_gdf['closest_ivt_time']
mask_time = lsoa_data_gdf['unit_time_diff'] >= 84.8
len(lsoa_data_gdf.loc[mask_time])
359
There are more LSOAs in this list than there were in the outliers list.
Plot the new flagged LSOAs next to the marked outliers:
# Plot data
fig, ax = plt.subplots(figsize=(8, 10))
lsoa_data_gdf.loc[mask_time].plot(
ax=ax, # Set which axes to use for plot (only one here)
color='magenta',
antialiased=False, # Avoids artifact boundry lines
edgecolor='face', # Make LSOA boundry same colour as area
)
mask = gdf_outliers[f'outlier_{col}'] == True
print(len(gdf_outliers.loc[mask]))
gdf_outliers.loc[mask].plot(
ax=ax, # Set which axes to use for plot (only one here)
color='LimeGreen',
antialiased=False, # Avoids artifact boundry lines
edgecolor='face', # Make LSOA boundry same colour as area
)
# Add country border
outline.plot(ax=ax, edgecolor='k', facecolor='None', linewidth=1.0)
plt.show()
266
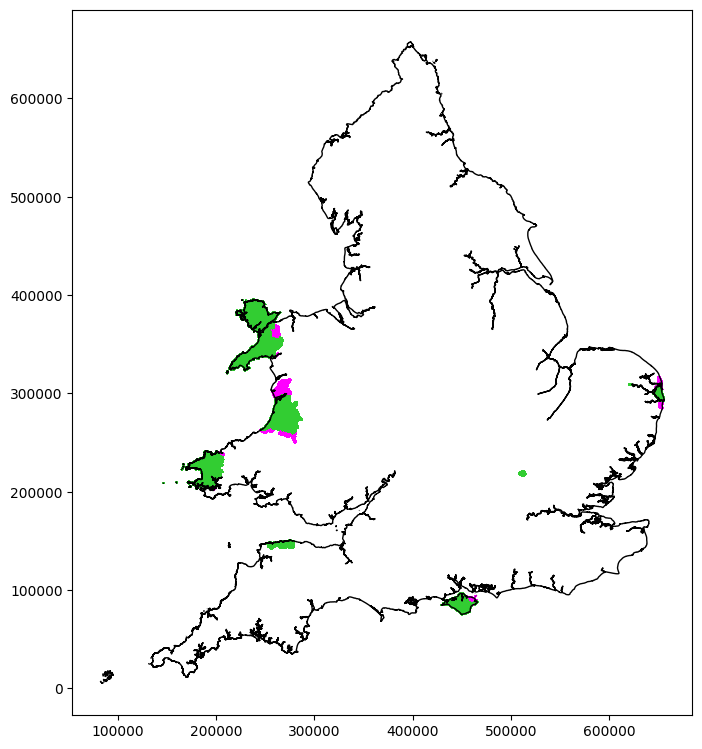
Generic geography#
Show two cases with the same circle plots as used in the generic geography example. The first case is the stroke units whose outliers have the smallest time difference between hospitals. The second case is the same for the biggest time difference.
# Take the mean of all values in the outlier dataframe grouped by closest IVT unit:
gdf_out_adv_mean = gdf_out_adv.groupby('closest_ivt_unit').mean(numeric_only=True)
# Only keep columns involving times:
gdf_out_adv_mean = gdf_out_adv_mean[
[t for t in gdf_out_adv.columns if (
('time' in t) | (t.endswith('drip_ship')) | (t.endswith('mothership'))
)]
]
# Sort by increasing time difference:
gdf_out_adv_mean = gdf_out_adv_mean.sort_values('unit_time_diff')
# Merge in stroke unit names:
gdf_out_adv_mean = pd.merge(
gdf_out_adv_mean, df_units[['stroke_team', 'region']],
left_index=True, right_index=True, how='left'
)
gdf_out_adv_mean
closest_ivt_time | closest_mt_time | transfer_mt_time | ivt_drip_ship | mt_drip_ship | ivt_mothership | mt_mothership | unit_time_diff | stroke_team | region | |
---|---|---|---|---|---|---|---|---|---|---|
closest_ivt_unit | ||||||||||
LU40DZ | 21.900000 | 91.700000 | 45.5 | 111.900000 | 277.400000 | 181.700000 | 241.700000 | 69.800000 | Luton and Dunstable Hospital | NHS Bedfordshire, Luton and Milton Keynes ICB ... |
EX314JB | 25.580000 | 117.720000 | 90.6 | 115.580000 | 326.180000 | 207.720000 | 267.720000 | 92.140000 | North Devon District Hospital, Barnstaple | NHS Devon ICB - 15N |
LL572PW | 25.594118 | 118.407059 | 103.5 | 115.594118 | 339.094118 | 208.407059 | 268.407059 | 92.812941 | Ysbyty Gwynedd (Bangor) | Betsi Cadwaladr University Health Board |
NR316LA | 13.147170 | 108.401887 | 105.7 | 103.147170 | 328.847170 | 198.401887 | 258.401887 | 95.254717 | James Paget Hospitals, Great Yarmouth | NHS Norfolk and Waveney ICB - 26A |
SA612PZ | 18.102857 | 116.385714 | 107.8 | 108.102857 | 335.902857 | 206.385714 | 266.385714 | 98.282857 | Withybush General Hospital (Haverfordwest) | Hywel Dda University Health Board |
PO305TG | 17.898485 | 122.633333 | 117.5 | 107.898485 | 345.398485 | 212.633333 | 272.633333 | 104.734848 | St Mary's Hospital, Newport | NHS Hampshire and Isle of Wight ICB - D9Y0V |
NR47UY | 12.200000 | 121.800000 | 78.8 | 102.200000 | 301.000000 | 211.800000 | 271.800000 | 109.600000 | Norfolk and Norwich University Hospital | NHS Norfolk and Waveney ICB - 26A |
SY231ER | 12.985000 | 133.985000 | 135.8 | 102.985000 | 358.785000 | 223.985000 | 283.985000 | 121.000000 | Bronglais Hospital (Aberystwyth) | Hywel Dda University Health Board |
The shortest time difference*: stroke unit EX31 4JB, North Devon District Hospital, Barnstaple.
The largest time difference: stroke unit SY23 1ER, Bronglais Hospital (Aberystwyth).
* excluding Central Bedfordshire with closest unit LU4 0DZ, where the times look a bit dodgy. The times are also fishy for Norwich - the combined travel to IVT and then MT unit is less than going directly to an MT unit.
Setup for outcomes#
import pandas as pd
import os
import matplotlib.pyplot as plt
import numpy as np
import sys
sys.path.insert(0, '../general_results')
from geography_calc import main
fixed_times = pd.read_csv(
os.path.join('..', 'england_wales', 'output', 'pathway_times.csv'),
index_col=0, header=None).squeeze()
patient_proportions = pd.read_csv(
os.path.join('..', 'england_wales', 'output', 'patient_proportions.csv'),
index_col=0, header=None).squeeze()
patient_proportions
0
haemorrhagic 0.13600
lvo_no_treatment 0.14648
lvo_ivt_only 0.00840
lvo_ivt_mt 0.08500
lvo_mt_only 0.01500
nlvo_no_treatment 0.50252
nlvo_ivt 0.10660
Name: 1, dtype: float64
Example 1:#
Calculate coordinates of an outlier patient:
gdf_patient = gdf_out_adv.loc[gdf_out_adv['closest_ivt_unit'] == 'EX314JB'].iloc[0]
time_to_ivt = gdf_patient['closest_ivt_time']
time_to_mt = gdf_patient['closest_mt_time']
time_transfer = gdf_patient['transfer_mt_time']
# Cosine rule:
ang = np.arccos(
(time_to_ivt**2.0 + time_transfer**2.0 - time_to_mt**2.0) /
(2 * time_to_ivt * time_transfer)
)
# Coordinates relative to IVT unit (sohcahtoa):
patient_x = time_to_ivt * np.sin(np.pi - ang)
patient_y = time_to_ivt * np.cos(np.pi - ang)
(patient_x, patient_y)
(17.13915576825811, 16.942825607064037)
# Add an extra fixed time that is specific to this generic geography example.
fixed_times['travel_ivt_to_mt'] = time_transfer
fixed_times['ivt_x'] = 0
fixed_times['ivt_y'] = 0
fixed_times['mt_x'] = 0
fixed_times['mt_y'] = -fixed_times['travel_ivt_to_mt']
# Calculate the fixed times to treatment _excluding_ the travel from the patient location to the first stroke unit.
# Times for usual care (the patient goes to their nearest stroke unit and is later transferred to the MT unit if necessary):
fixed_times['usual_care_ivt'] = (
fixed_times['onset_to_ambulance_arrival'] +
fixed_times['arrival_to_ivt']
)
fixed_times['usual_care_mt'] = (
fixed_times['onset_to_ambulance_arrival'] +
# fixed_times['arrival_to_ivt'] +
fixed_times['net_operational_delay_to_mt_for_transfer'] +
fixed_times['travel_ivt_to_mt'] +
fixed_times['arrival_to_mt']
)
# Times for mothership (the patient goes directly to the MT unit):
fixed_times['mothership_ivt'] = (
fixed_times['onset_to_ambulance_arrival'] +
fixed_times['arrival_to_ivt']
)
fixed_times['mothership_mt'] = (
fixed_times['onset_to_ambulance_arrival'] +
fixed_times['arrival_to_mt']
)
dict_grid_time_travel, dict_grid_time_travel_info, dict_outcomes_dicts = (
main(fixed_times, patient_proportions))
def plot_geography_outliers(dict_grid_time_travel, dict_grid_time_travel_info, dict_outcomes_dicts, patient_x, patient_y):
# Shared grid extents:
extent = [
-dict_grid_time_travel_info['diff']['mothership_grid_xy_max'],
dict_grid_time_travel_info['diff']['mothership_grid_xy_max'],
-dict_grid_time_travel_info['diff']['mothership_grid_xy_max'],
dict_grid_time_travel_info['diff']['mothership_grid_xy_max'],
]
# Shared colour limits:
vmin = min([
dict_outcomes_dicts['diff']['nlvo_ivt']['added_utility'].min(),
dict_outcomes_dicts['diff']['lvo']['added_utility'].min(),
dict_outcomes_dicts['diff']['mixed']['added_utility'].min(),
])
vmax = max([
dict_outcomes_dicts['diff']['nlvo_ivt']['added_utility'].max(),
dict_outcomes_dicts['diff']['lvo']['added_utility'].max(),
dict_outcomes_dicts['diff']['mixed']['added_utility'].max(),
])
vlim = max([abs(vmin), abs(vmax)])
vmin = -vlim
vmax = vlim
contour_levels = np.linspace(vmin, vmax, 9)
cmap = 'bwr'
fig, axs = plt.subplots(1, 4, figsize=(12, 4))
ax_time = axs[0]
ax_nlvo = axs[1]
ax_lvo = axs[2]
ax_mix = axs[3]
s = dict_grid_time_travel['diff'].shape
t = ax_time.imshow(
-dict_grid_time_travel['diff'],
extent=extent,
origin='lower'
)
plt.colorbar(t, orientation='horizontal', label='Travel time difference')
grid = dict_outcomes_dicts['diff']['nlvo_ivt']['added_utility'].values.reshape(s)
t = ax_nlvo.imshow(
grid,
extent=extent,
origin='lower',
vmin=vmin,
vmax=vmax,
cmap=cmap
)
plt.colorbar(t, orientation='horizontal', label='Added utility\n(advantage of mothership)')
CS = ax_nlvo.contour(
grid,
extent=extent,
levels=contour_levels,
colors='k',
linewidths=0.5
)
ax_nlvo.clabel(CS, inline=True, fontsize=10)
grid = dict_outcomes_dicts['diff']['lvo']['added_utility'].values.reshape(s)
t = ax_lvo.imshow(
grid,
extent=extent,
origin='lower',
vmin=vmin,
vmax=vmax,
cmap=cmap
)
plt.colorbar(t, orientation='horizontal', label='Added utility\n(advantage of mothership)')
CS = ax_lvo.contour(
grid,
extent=extent,
levels=contour_levels,
colors='k',
linewidths=0.5
)
ax_lvo.clabel(CS, inline=True, fontsize=10)
grid = dict_outcomes_dicts['diff']['mixed']['added_utility'].values.reshape(s)
t = ax_mix.imshow(
grid,
extent=extent,
origin='lower',
vmin=vmin,
vmax=vmax,
cmap=cmap
)
plt.colorbar(t, orientation='horizontal', label='Added utility\n(advantage of mothership)')
CS = ax_mix.contour(
grid,
extent=extent,
levels=contour_levels,
colors='k',
linewidths=0.5
)
ax_mix.clabel(CS, inline=True, fontsize=10)
for ax in axs:
ax.scatter(dict_grid_time_travel_info['diff']['usual_care_unit_x'], dict_grid_time_travel_info['diff']['usual_care_unit_y'], marker='o', color='k')
ax.scatter(dict_grid_time_travel_info['diff']['mothership_unit_x'], dict_grid_time_travel_info['diff']['mothership_unit_y'], marker='D', color='k')
ax.scatter(patient_x, patient_y, marker='x', color='k')
ax_time.set_title('Travel time')
ax_nlvo.set_title('nLVO')
ax_lvo.set_title('LVO')
ax_mix.set_title('mix')
plt.show()
plot_geography_outliers(dict_grid_time_travel, dict_grid_time_travel_info, dict_outcomes_dicts, patient_x, patient_y)
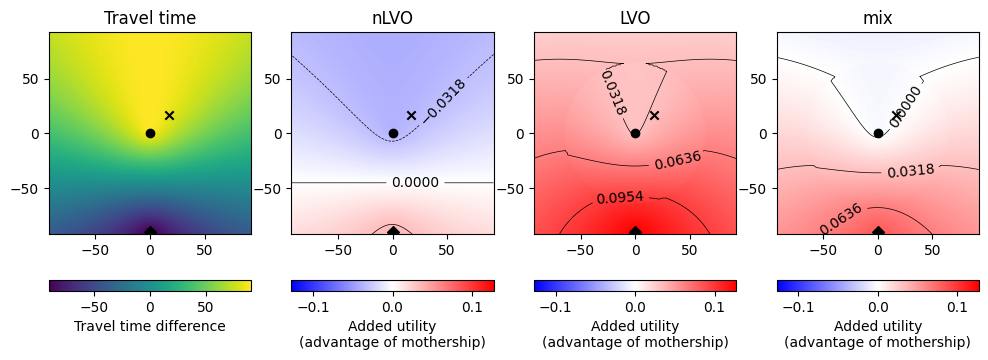
Utility matrix#
matrix_mixed = pd.read_csv('../general_results/output/outcome_matrix_treated_ischaemic.csv')
matrix_mixed
added_utility | mean_mrs | mrs_less_equal_2 | mrs_shift | onset_to_needle_mins | onset_to_puncture_mins | |
---|---|---|---|---|---|---|
0 | 0.204965 | 1.857484 | 0.641840 | -1.108209 | 0.0 | 0.0 |
1 | 0.203045 | 1.869066 | 0.639726 | -1.096627 | 10.0 | 0.0 |
2 | 0.201106 | 1.880724 | 0.637591 | -1.084969 | 20.0 | 0.0 |
3 | 0.199148 | 1.892456 | 0.635435 | -1.073237 | 30.0 | 0.0 |
4 | 0.197170 | 1.904261 | 0.633259 | -1.061432 | 40.0 | 0.0 |
... | ... | ... | ... | ... | ... | ... |
1857 | 0.007860 | 2.937686 | 0.430879 | -0.028007 | 330.0 | 480.0 |
1858 | 0.004365 | 2.956387 | 0.427194 | -0.009306 | 340.0 | 480.0 |
1859 | 0.000860 | 2.975102 | 0.423506 | 0.009409 | 350.0 | 480.0 |
1860 | -0.002655 | 2.993831 | 0.419814 | 0.028138 | 360.0 | 480.0 |
1861 | -0.006181 | 3.012572 | 0.416121 | 0.046879 | 370.0 | 480.0 |
1862 rows × 6 columns
n_times_ivt = len(set(matrix_mixed['onset_to_needle_mins']))
n_times_mt = len(set(matrix_mixed['onset_to_puncture_mins']))
grid_shape = (n_times_mt, n_times_ivt)
t_max_ivt = matrix_mixed['onset_to_needle_mins'].max()
t_max_mt = matrix_mixed['onset_to_puncture_mins'].max()
t_step_ivt = np.diff(matrix_mixed['onset_to_needle_mins'].values.reshape(grid_shape))[0][0]
t_step_mt = np.diff(matrix_mixed['onset_to_puncture_mins'].values.reshape(grid_shape))[0][0]
# Instead of the axes showing the row, column numbers of the grid,
# use this extent to scale the row, column numbers to the times.
# Extra division by 60 for conversion to hours.
grid_extent = np.array([
- t_step_ivt * 0.5, t_max_ivt + t_step_ivt * 0.5, # x-limits
- t_step_mt * 0.5, t_max_mt + t_step_mt * 0.5 # y-limits
]) / 60.0
import matplotlib.ticker as ticker # for axis tick locations
def plot_matrix_with_outliers(units_to_plot):
fig, ax = plt.subplots()
# Plot the matrix:
c = ax.imshow(
matrix_mixed['added_utility'].values.reshape(grid_shape),
extent=grid_extent,
origin='lower',
cmap='plasma'
)
plt.colorbar(c, label='Added utility')
# Contours:
img1 = ax.contour(
matrix_mixed['added_utility'].values.reshape(grid_shape),
extent=grid_extent,
levels=np.arange(0.0, 0.21, 0.01),
colors='k',
linewidths=0.5,
# aspect=aspect,
)
ax.clabel(img1, inline=True, fontsize=10)
for ivt_unit in units_to_plot:
gdf_patients_normal = lsoa_data_gdf.loc[lsoa_data_gdf['closest_ivt_unit'] == ivt_unit]
gdf_patients_outliers = gdf_out_adv.loc[gdf_out_adv['closest_ivt_unit'] == ivt_unit]
mask = gdf_patients_normal.index.isin(gdf_patients_outliers.index.values)
gdf_patients_normal = gdf_patients_normal.loc[~mask]
# Scatter some patient timings:
ax.scatter(
[gdf_patients_normal['ivt_drip_ship'] / 60.0, gdf_patients_normal['ivt_mothership'] / 60.0],
[gdf_patients_normal['mt_drip_ship'] / 60.0, gdf_patients_normal['mt_mothership'] / 60.0],
marker='x',
color='k',
label=f'{ivt_unit} normal'
# color='rgba(0, 0, 0, 0.2)'
)
ax.plot(
[gdf_patients_normal['ivt_drip_ship'] / 60.0, gdf_patients_normal['ivt_mothership'] / 60.0],
[gdf_patients_normal['mt_drip_ship'] / 60.0, gdf_patients_normal['mt_mothership'] / 60.0],
color='k',
)
# Outliers:
ax.scatter(
[gdf_patients_outliers['ivt_drip_ship'] / 60.0, gdf_patients_outliers['ivt_mothership'] / 60.0],
[gdf_patients_outliers['mt_drip_ship'] / 60.0, gdf_patients_outliers['mt_mothership'] / 60.0],
marker='x',
color='LimeGreen',
label=f'{ivt_unit} outliers'
# color='rgba(0, 0, 0, 0.2)'
)
ax.plot(
[gdf_patients_outliers['ivt_drip_ship'] / 60.0, gdf_patients_outliers['ivt_mothership'] / 60.0],
[gdf_patients_outliers['mt_drip_ship'] / 60.0, gdf_patients_outliers['mt_mothership'] / 60.0],
color='LimeGreen',
)
ax.legend()
ax.set_xlabel('Time to IVT (hours)\nif applicable')
ax.set_ylabel('Time to MT (hours)\nif applicable')
ax.xaxis.set_major_locator(ticker.MultipleLocator(1))
ax.xaxis.set_minor_locator(ticker.MultipleLocator(0.25))
ax.yaxis.set_major_locator(ticker.MultipleLocator(1))
ax.yaxis.set_minor_locator(ticker.MultipleLocator(0.25))
plt.show()
plot_matrix_with_outliers(['EX314JB'])
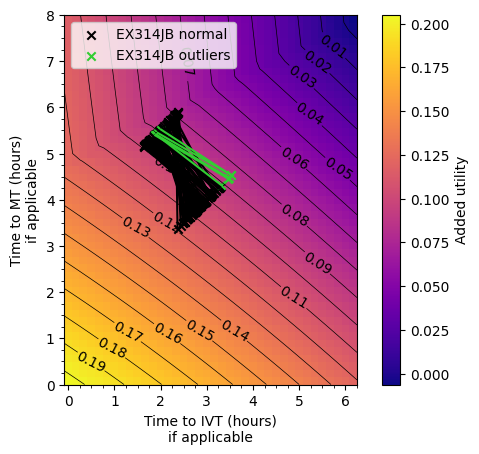
plot_matrix_with_outliers(['SY231ER'])
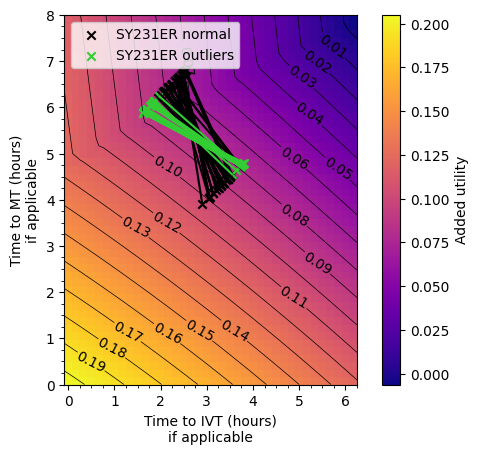
Conclusion#
The outlier areas are usually where there is a short travel time to the nearest stroke unit and a considerably longer time to the MT centre. The time difference is typically over 90 minutes.
The reason behind the worse outcomes is that the disbenefit from the increased time to IVT outweighs any additional benefit from decreasing time to MT.