Pathway patterns throughout the day
Contents
Pathway patterns throughout the day#
Aims#
Show key pathway statistics broken down by time of day (3 hour epochs).
Import libraries and data#
Data has been resticted to stroke teams with at least 300 admissions, with at least 10 patients receiving thrombolysis, over three years.
# import libraries
import matplotlib.pyplot as plt
import numpy as np
import pandas as pd
# Display entire dataframes
pd.set_option("display.max_rows", 999, "display.max_columns", 150)
# import data
raw_data = pd.read_csv(
'./../data/2019-11-04-HQIP303-Exeter_MA.csv', low_memory=False)
headings = list(raw_data)
print (headings)
['StrokeTeam', 'PatientUID', 'Pathway', 'S1AgeOnArrival', 'MoreEqual80y', 'S1Gender', 'S1Ethnicity', 'S1OnsetInHospital', 'S1OnsetToArrival_min', 'S1OnsetDateType', 'S1OnsetTimeType', 'S1ArriveByAmbulance', 'S1AdmissionHour', 'S1AdmissionDay', 'S1AdmissionQuarter', 'S1AdmissionYear', 'CongestiveHeartFailure', 'Hypertension', 'AtrialFibrillation', 'Diabetes', 'StrokeTIA', 'AFAntiplatelet', 'AFAnticoagulent', 'AFAnticoagulentVitK', 'AFAnticoagulentDOAC', 'AFAnticoagulentHeparin', 'S2INR', 'S2INRHigh', 'S2INRNK', 'S2NewAFDiagnosis', 'S2RankinBeforeStroke', 'Loc', 'LocQuestions', 'LocCommands', 'BestGaze', 'Visual', 'FacialPalsy', 'MotorArmLeft', 'MotorArmRight', 'MotorLegLeft', 'MotorLegRight', 'LimbAtaxia', 'Sensory', 'BestLanguage', 'Dysarthria', 'ExtinctionInattention', 'S2NihssArrival', 'S2BrainImagingTime_min', 'S2StrokeType', 'S2Thrombolysis', 'Haemorrhagic', 'TimeWindow', 'Comorbidity', 'Medication', 'Refusal', 'Age', 'Improving', 'TooMildSevere', 'TimeUnknownWakeUp', 'OtherMedical', 'S2ThrombolysisTime_min', 'S2TIAInLastMonth']
Restrict original data to hospitals with at least 300 admissions + 10 thrombolysis patients#
keep = []
groups = raw_data.groupby('StrokeTeam') # creates a new object of groups of data
for index, group_df in groups: # each group has an index and a dataframe of data
# Skip if total admissions less than 300 or total thrombolysis < 10
admissions = group_df.shape[0]
thrombolysis_received = group_df['S2Thrombolysis'] == 'Yes'
if (admissions < 300) or (thrombolysis_received.sum() < 10):
continue
else:
keep.append(group_df)
# Concatenate output
data = pd.DataFrame()
data = pd.concat(keep)
Remove in-hospital onset
mask = data['S1OnsetInHospital'] == 'No'
data = data[mask]
Group by time of day#
# Work on copy of data
data_time = data.copy()
# Set up results DataFrame
time_summary = pd.DataFrame()
# Count arrivals
time_summary['Arrivals'] = \
data_time.groupby('S1AdmissionHour').count()['StrokeTeam']
# Get thrombolysis rate
thrombolysed = data_time['S2Thrombolysis'] == 'Yes'
data_time['thrombolysed'] = thrombolysed
time_summary['thrombolyse_all'] = \
data_time.groupby('S1AdmissionHour').mean()['thrombolysed']
# Get proportion of strokes with known onset
onset_known = (data_time['S1OnsetTimeType'] == 'Best estimate') | \
(data_time['S1OnsetTimeType'] == 'Precise')
data_time['onset_known'] = onset_known
time_summary['onset_known'] = \
data_time.groupby('S1AdmissionHour').mean()['onset_known']
# Get proportion over 80
data_time['age_80_plus'] = data_time['MoreEqual80y'] == 'Yes'
time_summary['age_80_plus'] = \
data_time.groupby('S1AdmissionHour').mean()['age_80_plus']
# Get Rankin score
time_summary['rankin_all'] = \
data_time.groupby('S1AdmissionHour').mean()['S2RankinBeforeStroke']
# Get NIHSS
time_summary['nihss_all'] = \
data_time.groupby('S1AdmissionHour').mean()['S2NihssArrival']
# Get onset to arrival <4hrs and then restrict data
data_time['4hr_arrival'] = data_time['S1OnsetToArrival_min'] <= 240
time_summary['4hr_arrival'] = \
data_time.groupby('S1AdmissionHour').mean()['4hr_arrival']
mask = data_time['4hr_arrival']
data_time = data_time[mask]
# Get Rankin score of arrivals within 4hrs onset
time_summary['rankin_4hr'] = \
data_time.groupby('S1AdmissionHour').mean()['S2RankinBeforeStroke']
# Get NIHSS of arrivals within 4hrs onset
time_summary['nihss_4hr'] = \
data_time.groupby('S1AdmissionHour').mean()['S2NihssArrival']
# Get onset to arrival (of those arriving within 4 hours)
time_summary['onset_arrival'] = \
data_time.groupby('S1AdmissionHour').mean()['S1OnsetToArrival_min']
# Get scan in four hours (and remove rest)
data_time['4hr_scan'] = data_time['S2BrainImagingTime_min'] <= 240
time_summary['scan_4hrs'] = \
data_time.groupby('S1AdmissionHour').mean()['4hr_scan']
mask = data_time['4hr_scan']
data_time = data_time[mask]
# Get arrival to scan (of those arriving within 4 hours and scanned in 4hrs)
time_summary['arrival_scan'] = \
data_time.groupby('S1AdmissionHour').mean()['S2BrainImagingTime_min']
# Filter down to acanned within 4 hrs onset
onset_to_scan = (
data_time['S1OnsetToArrival_min'] + data_time['S2BrainImagingTime_min'])
data_time['onset_to_scan'] = onset_to_scan
mask = data_time['onset_to_scan'] <= 240
data_time = data_time[mask]
# Get thrombolysis given and remove rest
time_summary['thrombolyse_4hr'] = \
data_time.groupby('S1AdmissionHour').mean()['thrombolysed']
mask = data_time['thrombolysed']
# Get scan to needle
scan_to_needle = (
data_time['S2ThrombolysisTime_min'] - data_time['S2BrainImagingTime_min'])
data_time['scan_needle'] = scan_to_needle
time_summary['scan_to_needle'] = \
data_time.groupby('S1AdmissionHour').mean()['scan_needle']
# Get arrival to needle
time_summary['arrival_to_needle'] = \
data_time.groupby('S1AdmissionHour').mean()['S2ThrombolysisTime_min']
# Get onset to needle
onset_to_needle = (
data_time['S1OnsetToArrival_min'] + data_time['S2ThrombolysisTime_min'])
data_time['onset_to_needle'] = onset_to_needle
time_summary['onset_to_needle'] = \
data_time.groupby('S1AdmissionHour').mean()['onset_to_needle']
# Add short time start (string) column for plots
hour = ['0', '3', '6', '9', '12', '15', '19', '21']
time_summary['hour'] = hour
Show summary table by time of day#
time_summary.T
S1AdmissionHour | 00:00 to 02:59 | 03:00 to 05:59 | 06:00 to 08:59 | 09:00 to 11:59 | 12:00 to 14:59 | 15:00 to 17:59 | 18:00 to 20:59 | 21:00 to 23:59 |
---|---|---|---|---|---|---|---|---|
Arrivals | 9890 | 7367 | 15871 | 52049 | 48687 | 39838 | 32200 | 20918 |
thrombolyse_all | 0.131446 | 0.0651554 | 0.109004 | 0.119464 | 0.118882 | 0.115945 | 0.119658 | 0.135338 |
onset_known | 0.75996 | 0.679381 | 0.636633 | 0.63006 | 0.646004 | 0.658241 | 0.708571 | 0.75251 |
age_80_plus | 0.378564 | 0.379938 | 0.387562 | 0.436589 | 0.423768 | 0.414027 | 0.411677 | 0.412037 |
rankin_all | 1.02053 | 1.0566 | 1.05003 | 1.07894 | 1.03551 | 1.02995 | 1.03165 | 1.06062 |
nihss_all | 7.94191 | 8.35454 | 8.80986 | 7.71318 | 7.0694 | 6.80621 | 7.38032 | 7.62235 |
4hr_arrival | 0.464307 | 0.353197 | 0.388066 | 0.378778 | 0.36938 | 0.365304 | 0.423137 | 0.46362 |
rankin_4hr | 0.93162 | 1.04343 | 1.01153 | 1.13178 | 1.06611 | 1.02185 | 1.07134 | 1.03722 |
nihss_4hr | 9.47393 | 9.53222 | 10.0548 | 9.30759 | 8.91874 | 8.91479 | 9.32159 | 9.1475 |
onset_arrival | 122.127 | 115.238 | 102.11 | 108.779 | 113.879 | 111.436 | 110.78 | 112.673 |
scan_4hrs | 0.922474 | 0.895465 | 0.958597 | 0.95861 | 0.95863 | 0.954786 | 0.939523 | 0.926892 |
arrival_scan | 40.8057 | 47.2502 | 44.7102 | 41.357 | 42.0989 | 40.9557 | 42.8849 | 41.8893 |
thrombolyse_4hr | 0.334958 | 0.223671 | 0.310397 | 0.360533 | 0.373564 | 0.366626 | 0.333631 | 0.349285 |
scan_to_needle | 44.0687 | 44.2937 | 39.3118 | 31.7291 | 32.1522 | 33.7953 | 40.7675 | 44.3519 |
arrival_to_needle | 68.2312 | 68.0112 | 63.5706 | 53.7009 | 53.7034 | 55.1399 | 63.8725 | 69.2026 |
onset_to_needle | 177.516 | 175.269 | 150.697 | 146.731 | 150.852 | 149.811 | 158.123 | 165.162 |
hour | 0 | 3 | 6 | 9 | 12 | 15 | 19 | 21 |
Show summary charts of key metrics by time of day#
# Set up figure
fig = plt.figure(figsize=(12,15))
# Subplot 1: Arrivals
ax1 = fig.add_subplot(4,4,1)
x = time_summary['hour']
y = time_summary['Arrivals'] / time_summary['Arrivals'].sum() * 8
ax1.plot(x,y)
# Add line at 1
y1 = np.repeat(1,8)
ax1.plot(x,y1, color='0.5', linestyle=':')
ax1.set_ylim(ymin=0) # must be after plot method
ax1.set_xlabel('Time of day')
ax1.set_ylabel('Normlalised arrivals')
ax1.set_title('Arrivals\n(normalised to average)')
# Subplot 2: Thrombolysis
ax2 = fig.add_subplot(4,4,2)
x = time_summary['hour']
y = time_summary['thrombolyse_all'] * 100
ax2.plot(x,y)
ax2.set_xlabel('Time of day')
ax2.set_ylabel('Thrombolysis (%)')
ax2.set_title('Thrombolysis use\n(all arrivals)')
# Subplot 3: Known onset
ax3 = fig.add_subplot(4,4,3)
x = time_summary['hour']
y = time_summary['onset_known'] * 100
ax3.plot(x,y)
ax3.set_xlabel('Time of day')
ax3.set_ylabel('Onset known (%)')
ax3.set_title('Proportion with known onset')
# Subplot 4: age_80_plus
ax4 = fig.add_subplot(4,4,4)
x = time_summary['hour']
y = time_summary['age_80_plus'] * 100
ax4.plot(x,y)
ax4.set_xlabel('Time of day')
ax4.set_ylabel('Aged 80+')
ax4.set_title('Proportion aged 80+')
# Subplot 5: Rankin (all arrivals)
ax5 = fig.add_subplot(4,4,5)
x = time_summary['hour']
y = time_summary['rankin_all']
ax5.plot(x,y)
ax5.set_xlabel('Time of day')
ax5.set_ylabel('Rankin (mean)')
ax5.set_title('Mean pre-stroke\nmodified Rankin\n(all arrivals)')
# Subplot 6: NIHSS (all arrivals)
ax6 = fig.add_subplot(4,4,6)
x = time_summary['hour']
y = time_summary['nihss_all']
ax6.plot(x,y)
ax6.set_xlabel('Time of day')
ax6.set_ylabel('NIHSS (mean)')
ax6.set_title('Mean NIH Stroke Scale\n(all arrivals)')
# Subplot 7: 4hr_arrival
ax7 = fig.add_subplot(4,4,7)
x = time_summary['hour']
y = time_summary['4hr_arrival'] * 100
ax7.plot(x,y)
ax7.set_xlabel('Time of day')
ax7.set_ylabel('Arrive within 4hrs of onset (%)')
ax7.set_title('Proportion arriving within\n4hrs of known onset')
# Subplot 8: Rankin (4hr arrivals)
ax8 = fig.add_subplot(4,4,8)
x = time_summary['hour']
y = time_summary['rankin_4hr']
ax8.plot(x,y)
ax8.set_xlabel('Time of day')
ax8.set_ylabel('Rankin (mean)')
ax8.set_title('Mean pre-stroke\nmodified Rankin\n(arrivals 4hrs from onset)')
# Subplot 9: NIHSS (4hr arrivals)
ax9 = fig.add_subplot(4,4,9)
x = time_summary['hour']
y = time_summary['nihss_4hr']
ax9.plot(x,y)
ax9.set_xlabel('Time of day')
ax9.set_ylabel('NIHSS (mean)')
ax9.set_title('Mean NIH Stroke Scale\n(arrivals 4hrs from onset)')
# Subplot 10: onset_arrival (4hr arrivals)
ax10 = fig.add_subplot(4,4,10)
x = time_summary['hour']
y = time_summary['onset_arrival']
ax10.plot(x,y)
ax10.set_xlabel('Time of day')
ax10.set_ylabel('Onset to arrival (minutes, mean)')
ax10.set_title('Mean onset to arrival\n(arrivals 4hrs from onset)')
# Subplot 11: scan_4hrs (4hr arrivals)
ax11 = fig.add_subplot(4,4,11)
x = time_summary['hour']
y = time_summary['scan_4hrs'] * 100
ax11.plot(x,y)
ax11.set_xlabel('Time of day')
ax11.set_ylabel('Proportion scanned within 4hrs (%)')
ax11.set_title('Proportion scanned within\n4hrs of arrival\n(arrivals 4hrs from onset)')
# Subplot 12: arrival_scan (4hr scan)
ax12 = fig.add_subplot(4,4,12)
x = time_summary['hour']
y = time_summary['arrival_scan']
ax12.plot(x,y)
ax12.set_xlabel('Time of day')
ax12.set_ylabel('Arrival to scan (minutes, mean)')
ax12.set_title('Mean arrival to scan\n(scanned 4hrs from onset)')
# Subplot 13: thrombolysis (4hr scan)
ax13 = fig.add_subplot(4,4,13)
x = time_summary['hour']
y = time_summary['thrombolyse_4hr'] * 100
ax13.plot(x,y)
ax13.set_xlabel('Time of day')
ax13.set_ylabel('Thrombolsyis(%)')
ax13.set_title('Thrombolysis use\n(scanned 4hrs from onset)')
# Subplot 14: scan_to_needle
ax14 = fig.add_subplot(4,4,14)
x = time_summary['hour']
y = time_summary['scan_to_needle']
ax14.plot(x,y)
ax14.set_xlabel('Time of day')
ax14.set_ylabel('Scan to needle (minutes, mean)')
ax14.set_title('Mean scan to needle')
# Subplot 15: arrival_to_needle
ax15 = fig.add_subplot(4,4,15)
x = time_summary['hour']
y = time_summary['arrival_to_needle']
ax15.plot(x,y)
ax15.set_xlabel('Time of day')
ax15.set_ylabel('Arrival to needle (minutes, mean)')
ax15.set_title('Mean arrival to needle')
# Subplot 16: onset_to_needle
ax16 = fig.add_subplot(4,4,16)
x = time_summary['hour']
y = time_summary['onset_to_needle']
ax16.plot(x,y)
ax16.set_xlabel('Time of day')
ax16.set_ylabel('Onset to needle (minutes, mean)')
ax16.set_title('Mean onset to needle')
# Save and show
plt.tight_layout(pad=2)
plt.savefig('output/stats_by_time_of_day.jpg', dpi=300)
plt.show();
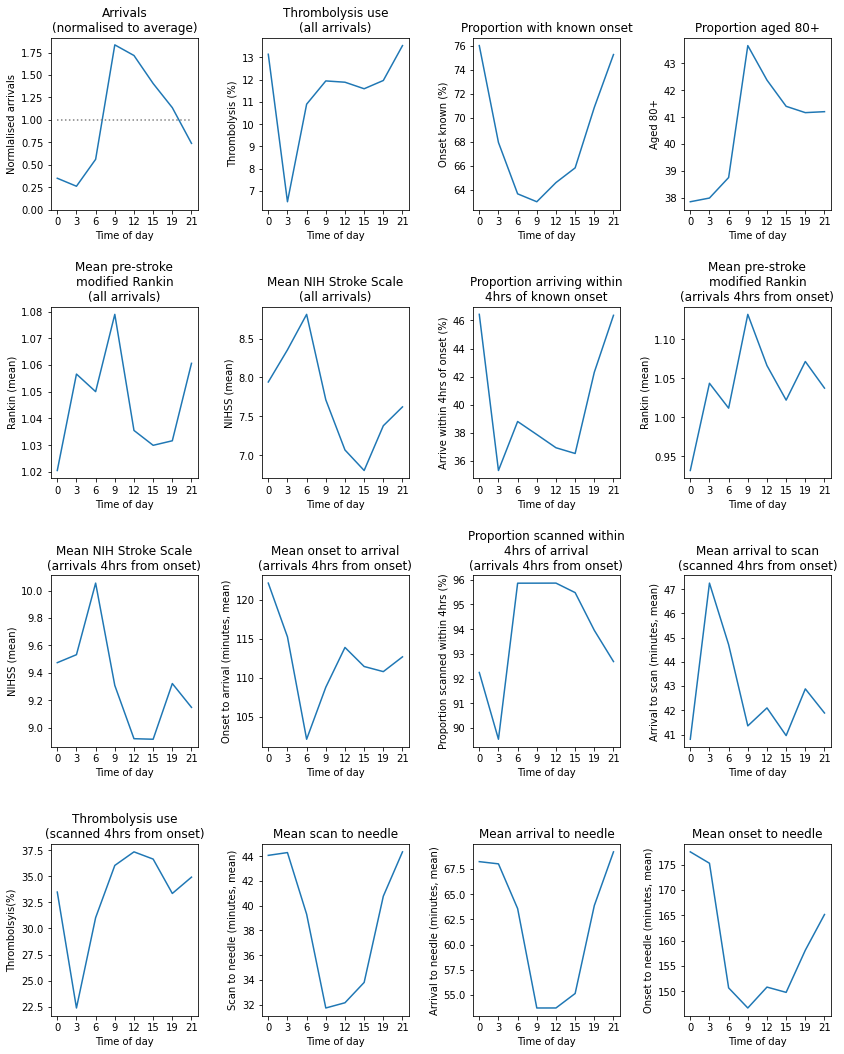
Observations#
Arrivals peak between 9am and 3pm, and then drop steadily to a low between 3am and 6pm.
Thrombolysis use is significantly lower in the 3am to 6am epoch, but only 3% of admissions occur in this period.
Arrival-to-scan time is higher 3am to 9am, but reasonably stable for the rest oif the day.
Scan-to-needle is approximately 10 minutes slower at night (9pm to 9am) than during the day (9am to 9pm).
The lowest proportion of arrivals with known stroke onset occurs during the day (6am to 6pm).