Plain English Summary (long)
Contents
Plain English Summary (long)#
“Your decision to treat or not treat … That’s the difficult part. That’s the grey area where everyone does a different thing.”
—Stroke Consultant during interviews for SAMueL
BACKGROUND AND SUMMARY
Stroke is a common cause of adult disability. Expert opinion is that about one in five patients should receive clot-busting drugs (‘thrombolysis’) to break up the blood clot that is causing their stroke. This is called thrombolysis. At the moment only about one in nine patients actually receive this treatment in the UK. There is a lot of variation between hospitals, which means that the same patient might receive different treatment in different hospitals.
Clot-busting drugs are not suitable for everyone. There is a small risk of a bleed in the brain. Doctors must feel confident in their use, and lack of confidence may explain some of the variation in use. In our work we have developed the methods for understanding what are the main causes of variation between hospitals: How much difference is due to processes (like how quickly a patient is scanned, an essential step), how much is due to differences in patient populations, and how much difference is due to different decision-making by doctors. This has enabled us to model the ideal number of patients who could or should be treated in each hospital in the UK. We predict that the number of people for whom clot-busting drugs would prevent disability after stroke could be nearly doubled.
WHAT WE DID
We did three things in the SAMueL project:
We built machine learning models that learned which patients would be given clot-busting drugs at each hospital. Machine learning works on the principle of asking and learning “What happened to similar patients in this hospital before?”
We built a simulation of stroke pathways for all hospitals. This simulation would pass individual patients through a pathway with each patient having their own unique speed of movement through the pathway, but with speeds all being typical of a particular hospital. This included patients that are typical of that hospital (matching, for example, the number of patients who arrive in hospital in time for clot-busting treatment). With this simulation we could ask questions like “What would happen if we could speed up the pathway at this hospital?”
We interviewed doctors about what we were doing. We asked for their feedback – was it easy to understand? Was it helpful to hear about how their patients would be treated at another hospital?
WHAT WE FOUND
Some of our most important findings were:
Our machine learning models were correct eight to nine times out of ten. They also report how sure they are about a decision, and the more sure they are the more often they are right.
Using our machine learning models we could answer the question “What treatment would this patient have likely received at different hospitals?”
Different hospitals would likely make different decisions on patients. Hospitals can more easily agree on who definitely should not be treated with clot-busting drugs than who should be.
There is large range in willingness to treat. Given the same set of patients, doctors who were most willing to treat would treat four to five times more patients than those least willing to treat.
If all hospitals made decisions like those of the top 30 most willing to treat hospitals, there would be 13 people treated for every 10 treated now. That would mean a 30% increase in the number of people without disability after their stroke because they received clot-busting treatment.
We can show individual hospitals examples of patients that they appear to treat differently from the top 30 most willing to treat hospitals. We hope this will help open up discussions on why different hospitals select different patients for treatment.
We can highlight patients who were not treated as we would expect them to be treated in the hospital that they attended.
Knowing the time a stroke started is vital if clot-busting drugs are to be given. Some hospitals do that for many more patients than others probably because they have worked out how to do this best, along with their local ambulance colleagues. If all hospitals managed to do as well as a ‘good’ hospital (about a quarter the way down the ‘league table’ of how many patients they determine the stroke onset time for), there would be 11-12 people treated for every 10 treated now, and more people leaving hospital without disability.
Before a patient can be treated they must have a brain scan, and then the treatment must be prepared and delivered. Hospitals manage to do this at different speeds. If all hospitals managed to do both of those tasks in 30 minutes (which some hospitals have shown is possible) then there would be 12-13 people treated for every 10 treated now. But as well as treating more patients, all patients would be treated more quickly, and that would mean a 50% increase in the number of people without disability after their stroke because they received clot-busting treatment.
If we combined all these changes across all hospitals then we would expect there would be 16 people treated for every 10 treated now. That is instead of treating about one in nine patients as now, we would treat one in five or six. More importantly, that would mean a 90% increase in the number of people without disability after their stroke because they received clot-busting treatment.
After we test all these changes at each hospital in our models we find there will still be quite a lot of variation in how many are treated at each hospital. This is because hospitals have different populations of patients. So rather than having a single target for treatment for all hospitals, it may be better to have a realistic target for each hospital, which takes their patient mix into account.
For each hospital we can predict which change would make most difference - is it speed, or willingness to treat, or knowing the stroke onset times? We can help hospitals identify what is best for them to work on improving, and that may be different to the hospital down the road.
Feedback from doctors throughout the project was really positive and useful. They helped us to work out what results to show and how to show them. They also had questions for us on how we could take this forward. They wanted to know how doctors would get to hear about our research. They wanted to know how we would communicate with managers and the people who pay for the hospitals if they needed to fund improvements in the service. Importantly, they wanted our machine learning models to learn to predict likely outcome for individuals, as well as the likely treatment decision. We also found that it was those doctors who were most experienced in this area who were the most open to how our work might help them improve treatment. That is reassuring, but it is actually those doctors who are less experienced in the use of clot-busting drugs that we want to reach most.
We are working with the ‘National Stroke Audit’ now on making our models part of their routine reporting back to hospitals. And we are, of course, working on what we shall do next to develop this work further!
Summary of findings#
Figure 4 shows a summary of the percieved problem and what we found by using modelling the pathway and the clinical decision-making.
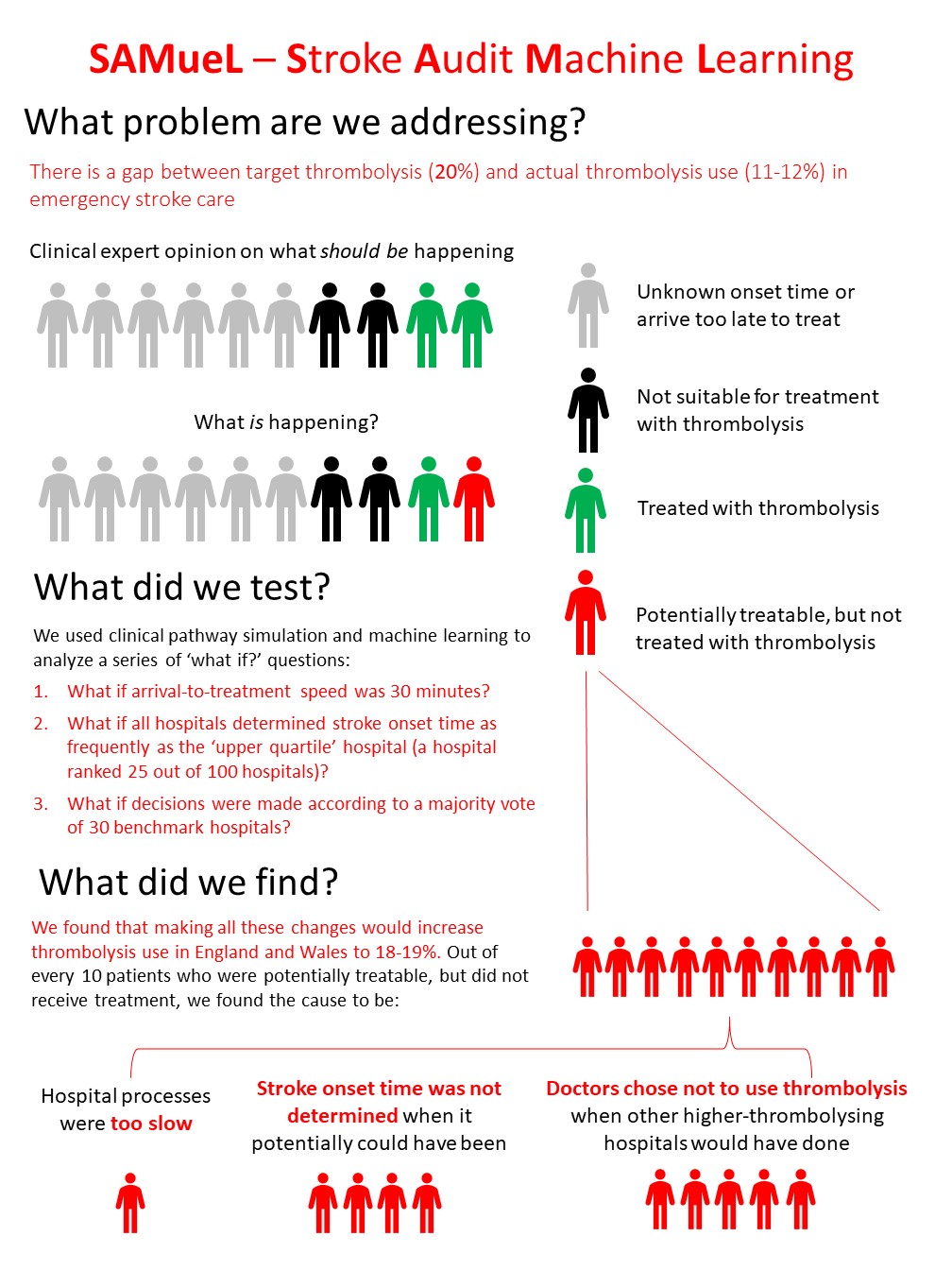
Fig. 4 A summary of the perceived problem and our findings. Clinical expert opinion is that two in every ten emergency stroke patients could be treated with thrombolysis, but the reality is that only about one in ten are treated with thrombolysis. This leaves about one in ten patients who could be treated, but are not. For patients who might be treated but were not, we found the main cause is likely to be variation in clinical decision-making between hospitals. After decision-making the change that would make the most difference was increasing the proportion of patients with known stroke onset time, to be at least the current national upper quartile. Finally speed increases would improve the use of thrombolysis a little (but would also benefit everyone who does receive thrombolysis, as the faster the treatment the better the likely outcome).#