How much of the inter-hospital variation in thrombolysis use do in-hospital processes explain
Contents
How much of the inter-hospital variation in thrombolysis use do in-hospital processes explain#
Aims:
Investigate the correlation (explained variance) between hospital model process parameters and the variation in use of thrombolysis between hospitals.
Load data and pivot by scenario#
import matplotlib.pyplot as plt
import numpy as np
import pandas as pd
# Load data
scenarios = pd.read_csv('output/key_scenario_results.csv')
# Performance data
performance = pd.read_csv(
'hosp_performance_output/hospital_performance.csv', index_col='stroke_team')
performance['hosp_speed'] = (
np.exp(performance['arrival_scan_arrival_mins_mu']) +
np.exp(performance['scan_needle_mins_mu']))
# Decision data
decision = pd.read_csv(
'../random_forest/predictions/corhort_rates.csv', index_col='hospital')
scenarios.head()
stroke_team | scenario | admissions | thrombolysis_rate | additional_good_outcomes_per_1000_patients | patients_receiving_thrombolysis | add_good_outcomes | |
---|---|---|---|---|---|---|---|
0 | AGNOF1041H | base | 671.666667 | 15.11 | 12.72 | 101.488833 | 8.543600 |
1 | AKCGO9726K | base | 1143.333333 | 15.06 | 13.43 | 172.186000 | 15.354967 |
2 | AOBTM3098N | base | 500.666667 | 7.81 | 5.74 | 39.102067 | 2.873827 |
3 | APXEE8191H | base | 439.333333 | 10.08 | 7.35 | 44.284800 | 3.229100 |
4 | ATDID5461S | base | 275.666667 | 9.20 | 6.42 | 25.361333 | 1.769780 |
performance.head()
thrombolysis_rate | admissions | 80_plus | onset_known | known_arrival_within_4hrs | onset_arrival_mins_mu | onset_arrival_mins_sigma | scan_within_4_hrs | arrival_scan_arrival_mins_mu | arrival_scan_arrival_mins_sigma | onset_scan_4_hrs | eligable | scan_needle_mins_mu | scan_needle_mins_sigma | hosp_speed | |
---|---|---|---|---|---|---|---|---|---|---|---|---|---|---|---|
stroke_team | |||||||||||||||
AGNOF1041H | 0.154839 | 671.666667 | 0.425459 | 0.635236 | 0.681250 | 4.576874 | 0.557598 | 0.965596 | 1.665700 | 1.497966 | 0.935867 | 0.388325 | 3.669602 | 0.664462 | 44.525658 |
AKCGO9726K | 0.158892 | 1143.333333 | 0.395658 | 0.970845 | 0.428829 | 4.625486 | 0.597451 | 0.955882 | 2.834183 | 0.999719 | 0.908425 | 0.419355 | 2.904479 | 0.874818 | 35.272215 |
AOBTM3098N | 0.085885 | 500.666667 | 0.485470 | 0.619174 | 0.629032 | 4.603918 | 0.584882 | 0.935043 | 3.471419 | 1.254744 | 0.846435 | 0.267819 | 3.694918 | 0.518929 | 72.424682 |
APXEE8191H | 0.098634 | 439.333333 | 0.515679 | 0.716237 | 0.608051 | 4.590357 | 0.496452 | 0.966899 | 3.312930 | 0.714465 | 0.904505 | 0.258964 | 3.585094 | 0.751204 | 63.522230 |
ATDID5461S | 0.090689 | 275.666667 | 0.533546 | 0.573156 | 0.660338 | 4.427826 | 0.591373 | 0.878594 | 4.125690 | 0.549301 | 0.865455 | 0.315126 | 3.497262 | 0.608126 | 94.935429 |
rx = scenarios.pivot(
index='stroke_team', columns='scenario', values='thrombolysis_rate')
rx = rx.merge(performance[['hosp_speed', 'onset_known']], left_index=True, right_index=True)
rx = rx.merge(decision['cohort_rate'], left_index=True, right_index=True)
rx.head()
base | benchmark | onset | onset_benchmark | same_patient_characteristics | speed | speed_benchmark | speed_onset | speed_onset_benchmark | hosp_speed | onset_known | cohort_rate | |
---|---|---|---|---|---|---|---|---|---|---|---|---|
AGNOF1041H | 15.11 | 20.38 | 18.17 | 24.09 | 11.04 | 15.21 | 20.14 | 17.89 | 23.90 | 44.525658 | 0.635236 | 27.76 |
AKCGO9726K | 15.06 | 14.18 | 14.92 | 14.27 | 22.22 | 15.32 | 14.69 | 15.36 | 14.83 | 35.272215 | 0.970845 | 37.45 |
AOBTM3098N | 7.81 | 11.88 | 9.39 | 14.52 | 8.74 | 9.39 | 13.78 | 11.40 | 17.17 | 72.424682 | 0.619174 | 26.00 |
APXEE8191H | 10.08 | 13.06 | 10.76 | 13.35 | 13.24 | 10.15 | 12.80 | 10.90 | 13.54 | 63.522230 | 0.716237 | 29.97 |
ATDID5461S | 9.20 | 9.92 | 11.81 | 13.35 | 7.60 | 11.10 | 11.79 | 14.64 | 15.95 | 94.935429 | 0.573156 | 25.92 |
Calculate difference between each hospital’s thrombolysis rate and the mean thrombolysis#
mean_rx = rx['base'].mean()
print (f'Mean thrombolysis: {mean_rx:0.2f}')
rx['diff_from_mean'] = rx['base'] - mean_rx
Mean thrombolysis: 11.22
rx.head()
base | benchmark | onset | onset_benchmark | same_patient_characteristics | speed | speed_benchmark | speed_onset | speed_onset_benchmark | hosp_speed | onset_known | cohort_rate | diff_from_mean | |
---|---|---|---|---|---|---|---|---|---|---|---|---|---|
AGNOF1041H | 15.11 | 20.38 | 18.17 | 24.09 | 11.04 | 15.21 | 20.14 | 17.89 | 23.90 | 44.525658 | 0.635236 | 27.76 | 3.889545 |
AKCGO9726K | 15.06 | 14.18 | 14.92 | 14.27 | 22.22 | 15.32 | 14.69 | 15.36 | 14.83 | 35.272215 | 0.970845 | 37.45 | 3.839545 |
AOBTM3098N | 7.81 | 11.88 | 9.39 | 14.52 | 8.74 | 9.39 | 13.78 | 11.40 | 17.17 | 72.424682 | 0.619174 | 26.00 | -3.410455 |
APXEE8191H | 10.08 | 13.06 | 10.76 | 13.35 | 13.24 | 10.15 | 12.80 | 10.90 | 13.54 | 63.522230 | 0.716237 | 29.97 | -1.140455 |
ATDID5461S | 9.20 | 9.92 | 11.81 | 13.35 | 7.60 | 11.10 | 11.79 | 14.64 | 15.95 | 94.935429 | 0.573156 | 25.92 | -2.020455 |
How much variation is explained by differences in decision making?#
diff_explained_by_decison_making = (np.corrcoef(
rx['cohort_rate'], rx['diff_from_mean'])[1,0]) ** 2
print(f'{diff_explained_by_decison_making:0.3}')
0.399
How much variation is explained by differences in speed?#
diff_explained_by_speed = (np.corrcoef(
rx['diff_from_mean'], rx['hosp_speed'])[1,0]) ** 2
print(f'{diff_explained_by_speed:0.3}')
0.254
How much variation is explained by determination of stroke onset time?#
diff_explained_by_determination_of_onset = (np.corrcoef(
rx['diff_from_mean'], rx['onset_known'])[1,0]) ** 2
print(f'{diff_explained_by_determination_of_onset:0.3}')
0.0474
Plot relationships#
from scipy import stats
fig = plt.figure(figsize=(15,6))
ax1 = fig.add_subplot(131)
x = rx['cohort_rate']
y = rx['diff_from_mean']
gradient, intercept, r_value, p_value, std_err = stats.linregress(x,y)
y_fit=intercept + (x*gradient)
plt.plot(x, y, 'o', label='original data')
plt.plot(x, y_fit, 'r', label='fitted line')
text='Slope: %.2f\nR-Squared: %.3f\nP value: %.3f' %(gradient,r_value**2,p_value)
plt.text(35, -8, text)
ax1.set_title('Relationship between thrombolysis use and\ndecision making')
ax1.set_xlabel('Predicted 10k cohort thrombolysis rate')
ax1.set_ylabel('Difference in thrombolysis from mean')
ax2 = fig.add_subplot(132)
x = rx['hosp_speed']
y = rx['diff_from_mean']
gradient, intercept, r_value, p_value, std_err = stats.linregress(x,y)
y_fit=intercept + (x*gradient)
plt.plot(x, y, 'o', label='original data')
plt.plot(x, y_fit, 'r', label='fitted line')
text='Slope: %.2f\nR-Squared: %.3f\nP value: %.3f' %(gradient,r_value**2,p_value)
plt.text(77, 10, text)
ax2.set_title('Relationship between thrombolysis use and\npathway speed')
ax2.set_xlabel('Arrival to scan + scan to needle (mins)')
ax2.set_ylabel('Difference in thrombolysis from mean')
ax3 = fig.add_subplot(133)
x = rx['onset_known']
y = rx['diff_from_mean']
gradient, intercept, r_value, p_value, std_err = stats.linregress(x,y)
y_fit=intercept + (x*gradient)
plt.plot(x, y, 'o', label='original data')
plt.plot(x, y_fit, 'r', label='fitted line')
text='Slope: %.2f\nR-Squared: %.3f\nP value: %.3f' %(gradient,r_value**2,p_value)
plt.text(0.38,10, text)
ax3.set_title('Relationship between thrombolysis use and\ndetermination of stroke onset')
ax3.set_xlabel('Proportion patients with determined stroke onset')
ax3.set_ylabel('Difference in thrombolysis from mean')
plt.tight_layout(pad=2)
plt.savefig('./output/model_correlations.jpg', dpi=300)
plt.show()
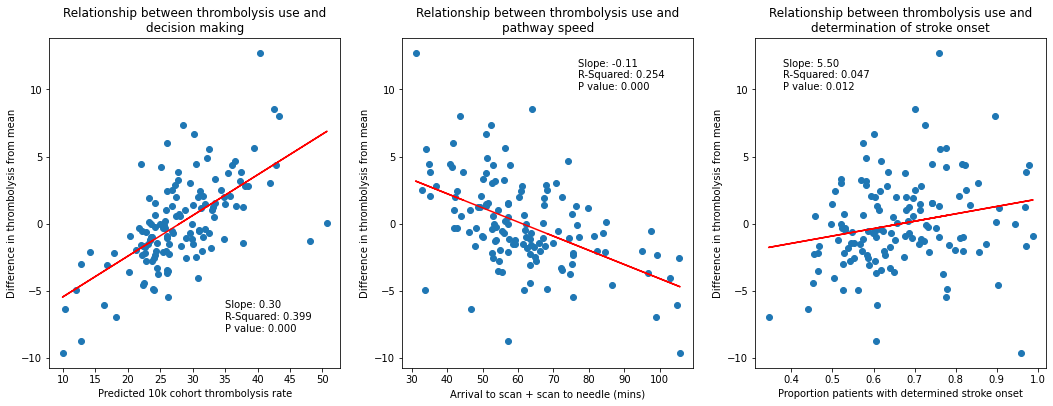
Conclusions#
In-hospital process parameters partly explain the inter-hospital variation in thrombolysis use.
The strongest relationship is between decision-making, as described by the predicted thrombolysis use of a standard 10k cohort of patients, with R-square of 0.40.
Pathway speed is the next strongest predictor of thrombolysis use, with an R-square of 0.25.
Determination of stroke onset time is the weakest predictor of thrombolysis use (R-square of 0.05), but is still statistically significant (p=0.012)